PixelFlow allows you to use all these features
Unlock the full potential of generative AI with Segmind. Create stunning visuals and innovative designs with total creative control. Take advantage of powerful development tools to automate processes and models, elevating your creative workflow.
Segmented Creation Workflow
Gain greater control by dividing the creative process into distinct steps, refining each phase.
Customized Output
Customize at various stages, from initial generation to final adjustments, ensuring tailored creative outputs.
Layering Different Models
Integrate and utilize multiple models simultaneously, producing complex and polished creative results.
Workflow APIs
Deploy Pixelflows as APIs quickly, without server setup, ensuring scalability and efficiency.
Stable Diffusion 1.5 Outpainting
Outpainting, also known as "generative fill", "Uncrop", or "Unlimited zoom", is the process of extending an image beyond its original borders, adding new elements in a consistent style or exploring new narrative paths. This model, with its unique capabilities, allows for the creation of surreal and expansive images, pushing the boundaries of traditional image generation.
On the technical side, Stable Diffusion 1.5 Outpainting employs a latent diffusion model that combines an autoencoder with a diffusion model trained in the autoencoder's latent space. The model uses an encoder to transform images into latent representations, with a relative downsampling factor of 8. Text prompts are processed through a ViT-L/14 text-encoder, and the non-pooled output of this encoder is fed into the UNet backbone of the latent diffusion model via cross-attention. The model's loss is a reconstruction objective between the added noise to the latent and the prediction made by the UNet. The strength value, which denotes the amount of noise added to the output image, can be adjusted to produce more variation within the image.
It allows users to break free from the 1:1 aspect ratio limitation of many generative model images, offering the freedom to create larger scenes and expand landscapes. Despite its surreal default nature, the model provides the flexibility to adjust the level of surrealism based on the user's preference. Moreover, it doesn't increase the image size infinitely but pushes the original image deeper into the canvas, mimicking the way cameras work when you take a few steps back.
Stable Diffusion 1.5 Outpainting use cases
-
Customized Digital Artwork: Artists can use the outpainting feature to create unique digital art pieces, expanding the canvas to add more elements and details. This can be particularly useful for creating panoramic landscapes or intricate scenes that require a larger canvas.
-
Film and Animation: In the film and animation industry, the outpainting feature can be used to extend scenes or backgrounds, providing a cost-effective alternative to manual drawing or CGI. This can be especially useful for creating wide-angle shots or panoramic views.
-
Advertising and Marketing: Marketers can use outpainting to adjust the aspect ratio of images to fit different advertising mediums. For instance, a square image can be outpainted to a landscape format for a billboard advertisement, or a portrait format for a mobile ad.
-
Game Design: In the gaming industry, outpainting can be used to generate diverse and expansive game environments. This can help game designers to quickly create new levels or scenes, saving time and resources.
-
Interior Design and Architecture: Outpainting can be used to visualize different design concepts or architectural plans. For example, an interior designer can use it to extend a room's image to see how it would look with additional elements or changes.
-
Fashion and Apparel Design: Designers can use outpainting to extend the design of a piece of clothing or an accessory, allowing them to visualize the complete look and make necessary adjustments.
-
Reimagining Historical or Classic Art: Artists can use outpainting to add a modern twist to historical or classic art pieces, extending the original artwork with new elements or styles.
Stable Diffusion 1.5 Outpainting license
The model is licensed under the Creative ML OpenRAIL-M license, a form of Responsible AI License (RAIL). This license prohibits certain use cases, including crime, libel, harassment, doxing, exploiting minors, giving medical advice, automatically creating legal obligations, producing legal evidence, and discrimination. However, users retain the rights to their generated output images and are free to use them commercially.
Other Popular Models
faceswap-v2
Take a picture/gif and replace the face in it with a face of your choice. You only need one image of the desired face. No dataset, no training
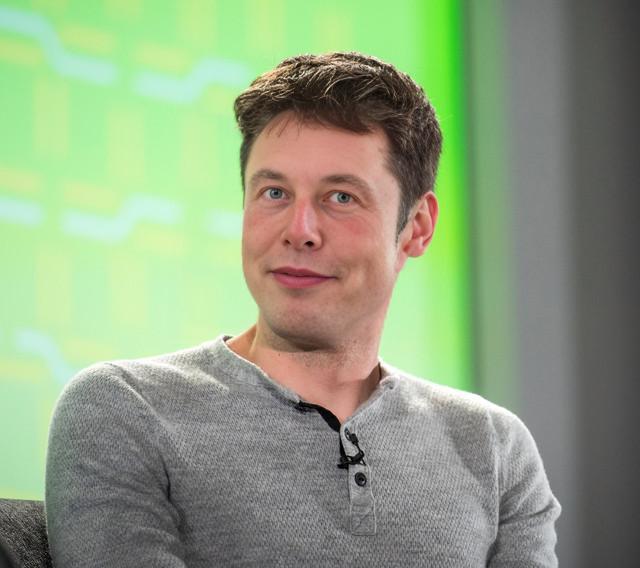
sdxl1.0-txt2img
The SDXL model is the official upgrade to the v1.5 model. The model is released as open-source software
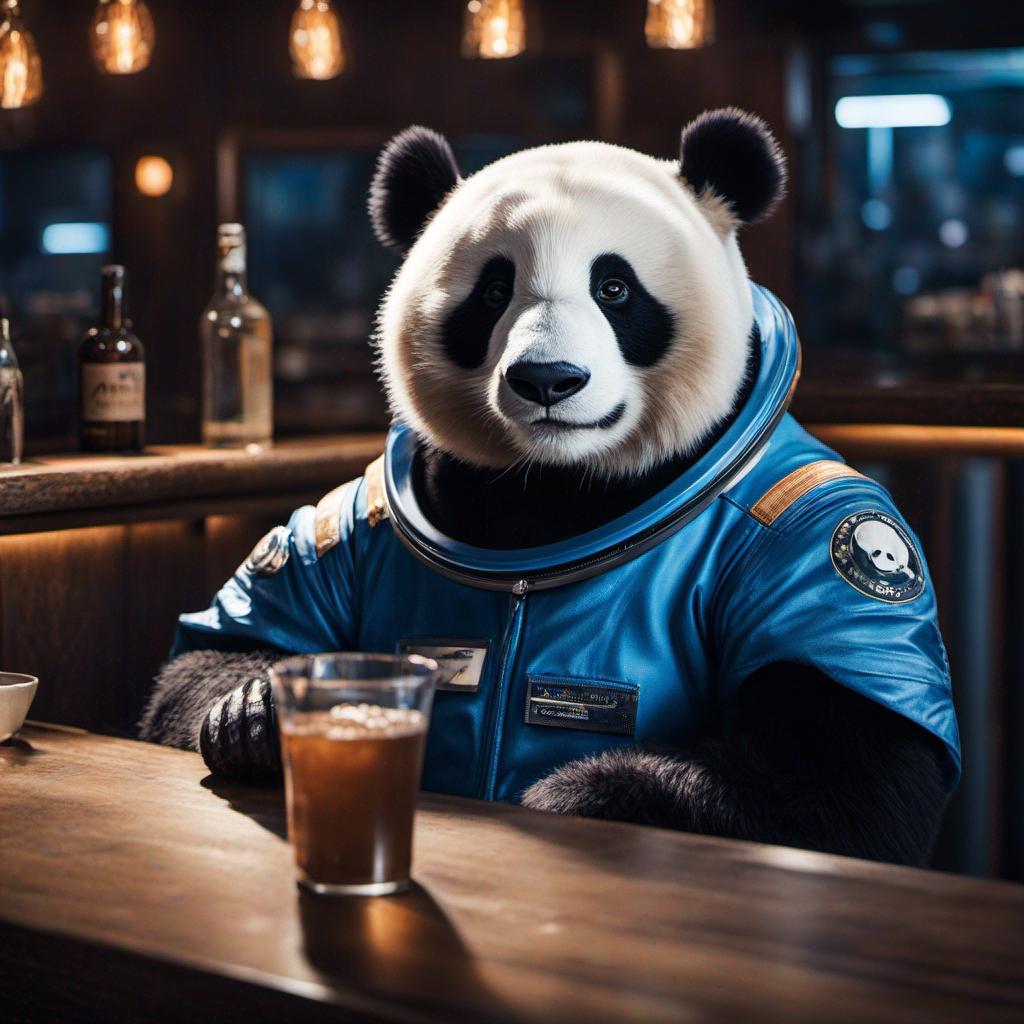
sd2.1-faceswapper
Take a picture/gif and replace the face in it with a face of your choice. You only need one image of the desired face. No dataset, no training
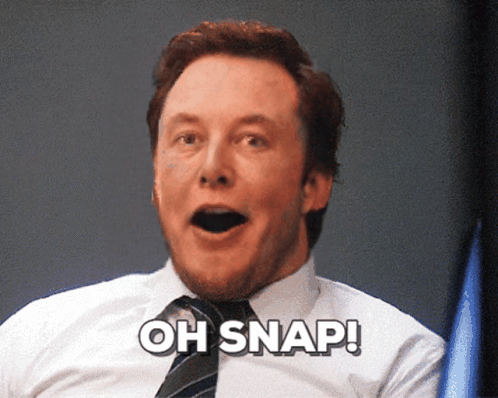
esrgan
ERGAN is an Image Super-Resolution (upscaler) model that enhances images with stunning, high-quality upscaling while preserving the exact composition of the original source. It improves detail without altering the image content.
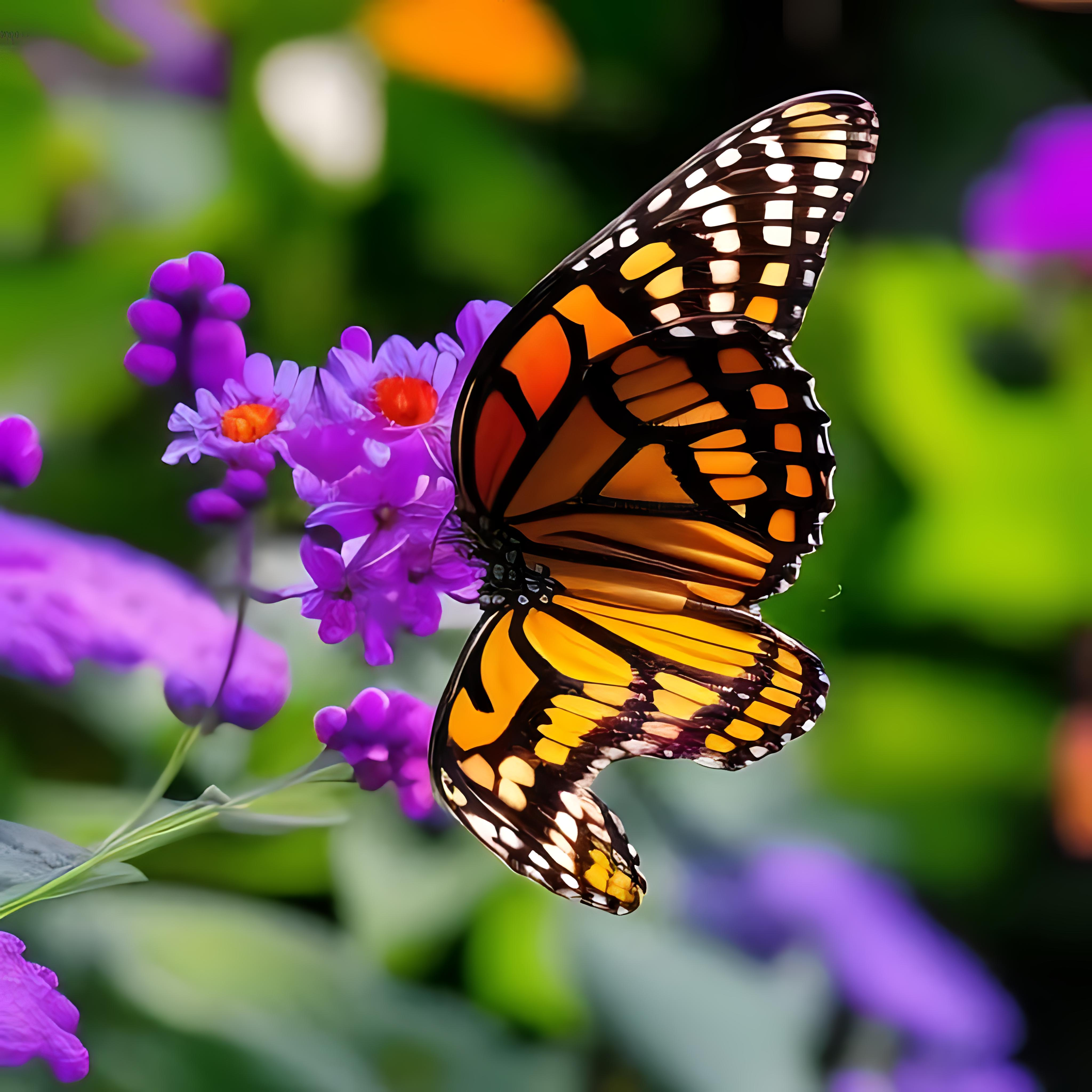