API
If you're looking for an API, you can choose from your desired programming language.
1
2
3
4
5
6
7
8
9
10
11
12
13
14
15
16
17
18
19
20
21
22
23
24
25
26
27
28
29
30
31
32
33
34
35
36
37
38
39
40
41
import requests
import base64
# Use this function to convert an image file from the filesystem to base64
def image_file_to_base64(image_path):
with open(image_path, 'rb') as f:
image_data = f.read()
return base64.b64encode(image_data).decode('utf-8')
# Use this function to fetch an image from a URL and convert it to base64
def image_url_to_base64(image_url):
response = requests.get(image_url)
image_data = response.content
return base64.b64encode(image_data).decode('utf-8')
# Use this function to convert a list of image URLs to base64
def image_urls_to_base64(image_urls):
return [image_url_to_base64(url) for url in image_urls]
api_key = "YOUR_API_KEY"
url = "https://api.segmind.com/v1/ostris-ikea-instructions-lora-sdxl"
# Request payload
data = {
"prompt": "Cyborg",
"negative_prompt": "boring, poorly drawn, bad artist, (worst quality:1.4), simple background, uninspired, (bad quality:1.4), monochrome, low background contrast, background noise, duplicate, crowded, (nipples:1.2), big breasts",
"scheduler": "UniPC",
"num_inference_steps": 25,
"guidance_scale": 8,
"samples": 1,
"seed": 3426017487,
"img_width": 1024,
"img_height": 1024,
"base64": False,
"lora_scale": 1
}
headers = {'x-api-key': api_key}
response = requests.post(url, json=data, headers=headers)
print(response.content) # The response is the generated image
Attributes
Prompt to render
Prompts to exclude, eg. 'bad anatomy, bad hands, missing fingers'
Type of scheduler.
Allowed values:
Number of denoising steps.
min : 20,
max : 100
Scale for classifier-free guidance
min : 0.1,
max : 25
Number of samples to generate.
min : 1,
max : 4
Seed for image generation.
Width of the image.
Allowed values:
Height of the Image
Allowed values:
Base64 encoding of the output image.
Scale of the lora
To keep track of your credit usage, you can inspect the response headers of each API call. The x-remaining-credits property will indicate the number of remaining credits in your account. Ensure you monitor this value to avoid any disruptions in your API usage.
Ikea Instructions LoRA SDXL
Ikea Instructions LoRA SDXL model is fine-tuned on IKEA diagrams. This model is designed to simplify and enhance the visual representation of furniture assembly instructions, making the process more intuitive and user-friendly. By training on a comprehensive collection of IKEA assembly diagrams, this model specializes in generating clear, concise, and easy-to-follow visual instructions.
Advantages
-
Enhanced Clarity in Instructions: Produces detailed and straightforward assembly guides.
-
Fine-Tuned on IKEA Diagram:Specifically trained for accuracy in furniture assembly visuals.
-
User-Friendly Visuals: Designed to make assembly processes more accessible and less daunting.
Use Cases
-
Furniture Assembly Guides:Ideal for creating or enhancing visual instructions for furniture assembly.
-
Product Design and Development: Assists designers in visualizing and communicating assembly steps.
-
Customer Support: Enhances customer experience by providing clearer assembly instructions.
-
Marketing and Sales: Can be used in promotional materials to demonstrate the ease of assembly.
Other Popular Models
faceswap-v2
Take a picture/gif and replace the face in it with a face of your choice. You only need one image of the desired face. No dataset, no training
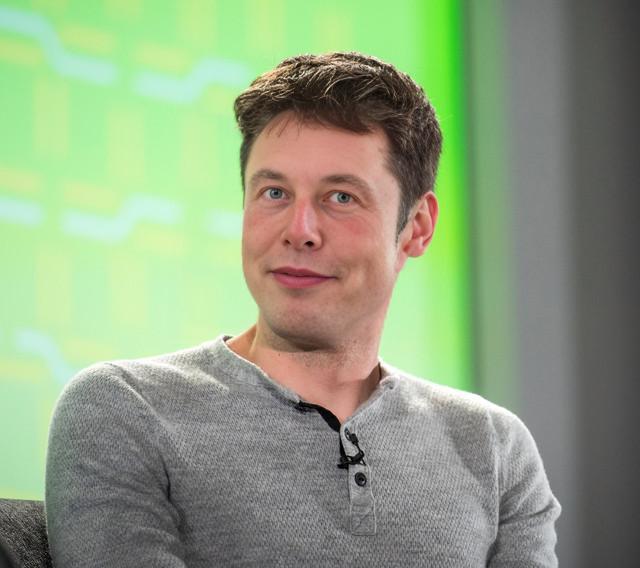
sdxl-inpaint
This model is capable of generating photo-realistic images given any text input, with the extra capability of inpainting the pictures by using a mask
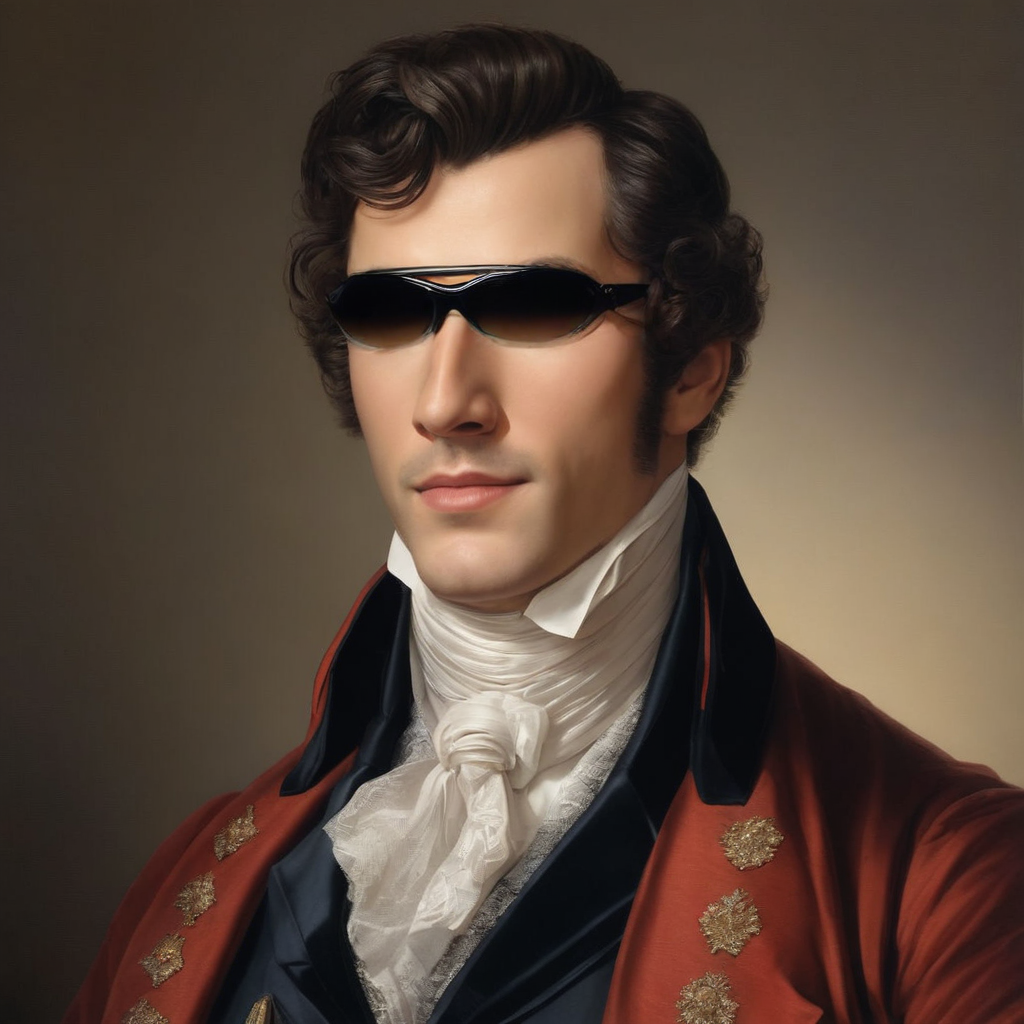
sdxl1.0-txt2img
The SDXL model is the official upgrade to the v1.5 model. The model is released as open-source software
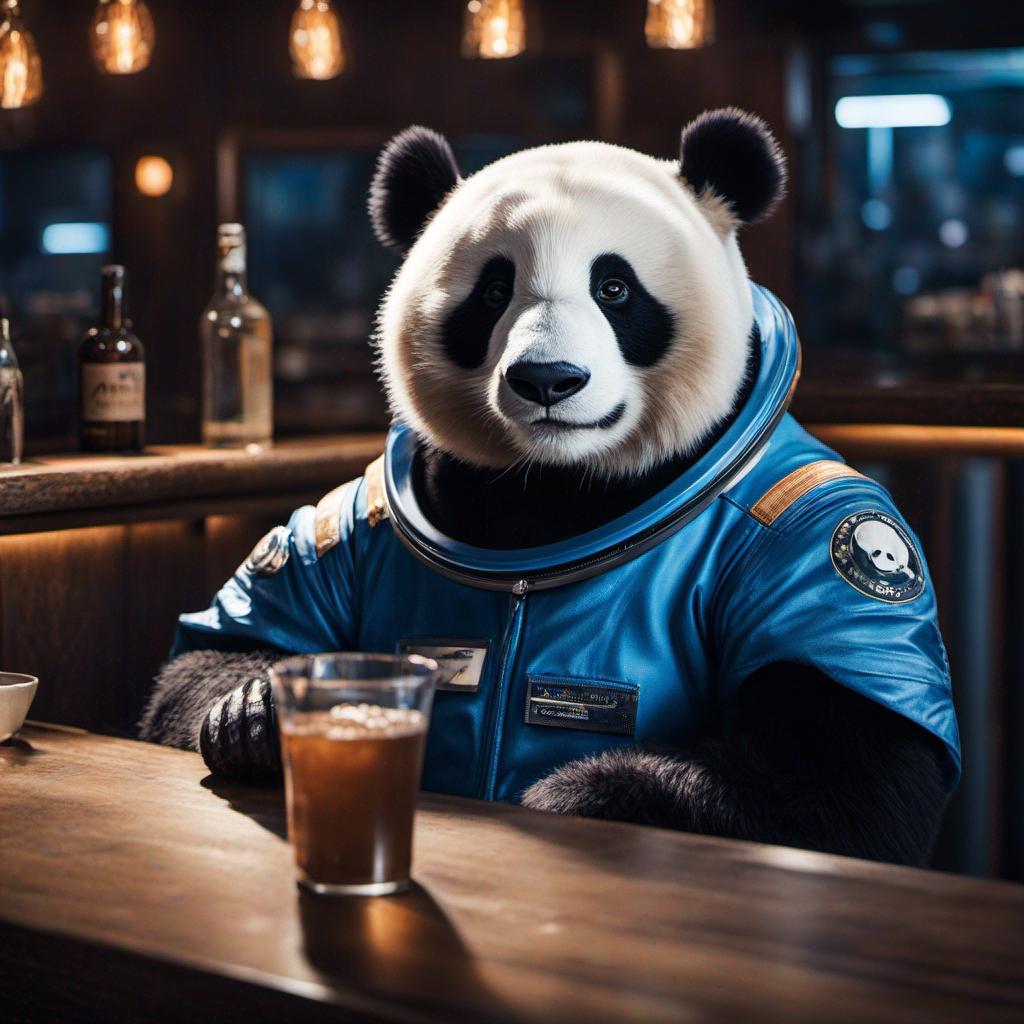
sd2.1-faceswapper
Take a picture/gif and replace the face in it with a face of your choice. You only need one image of the desired face. No dataset, no training
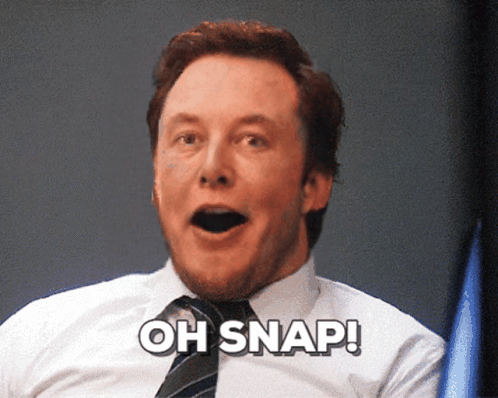