API
If you're looking for an API, you can choose from your desired programming language.
1
2
3
4
5
6
7
8
9
10
11
12
13
14
15
16
17
18
19
20
21
22
23
24
25
26
27
28
29
30
31
32
33
34
35
36
37
38
39
40
41
import requests
import base64
# Use this function to convert an image file from the filesystem to base64
def image_file_to_base64(image_path):
with open(image_path, 'rb') as f:
image_data = f.read()
return base64.b64encode(image_data).decode('utf-8')
# Use this function to fetch an image from a URL and convert it to base64
def image_url_to_base64(image_url):
response = requests.get(image_url)
image_data = response.content
return base64.b64encode(image_data).decode('utf-8')
# Use this function to convert a list of image URLs to base64
def image_urls_to_base64(image_urls):
return [image_url_to_base64(url) for url in image_urls]
api_key = "YOUR_API_KEY"
url = "https://api.segmind.com/v1/ostris-stained-glass-style-sdxl"
# Request payload
data = {
"prompt": "stained glass style, mickey mouse",
"negative_prompt": "boring, poorly drawn, bad artist, (worst quality:1.4), simple background, uninspired, (bad quality:1.4), monochrome, low background contrast, background noise, duplicate, crowded, (nipples:1.2), big breasts",
"scheduler": "UniPC",
"num_inference_steps": 25,
"guidance_scale": 8,
"samples": 1,
"seed": 3426017487,
"img_width": 1024,
"img_height": 1024,
"base64": False,
"lora_scale": 1
}
headers = {'x-api-key': api_key}
response = requests.post(url, json=data, headers=headers)
print(response.content) # The response is the generated image
Attributes
Prompt to render
Prompts to exclude, eg. 'bad anatomy, bad hands, missing fingers'
Type of scheduler.
Allowed values:
Number of denoising steps.
min : 20,
max : 100
Scale for classifier-free guidance
min : 0.1,
max : 25
Number of samples to generate.
min : 1,
max : 4
Seed for image generation.
Width of the image.
Allowed values:
Height of the Image
Allowed values:
Base64 encoding of the output image.
Scale of the lora
To keep track of your credit usage, you can inspect the response headers of each API call. The x-remaining-credits property will indicate the number of remaining credits in your account. Ensure you monitor this value to avoid any disruptions in your API usage.
Stained Glass Style SDXL
Stained Glass Style SDXL is trained extensively on diverse stained glass images, this model captures the intricate patterns, vibrant colors, and unique textures that define this ancient art form. The result is a powerful tool that can replicate the essence of stained glass in digital artworks.
Advantages
-
Authentic Replication: Accurately mimics the distinct characteristics of traditional stained glass.
-
Color Richness:Captures the vibrant and varied hues typical of stained glass art.
-
Detail and Texture: Reproduces the intricate details and textures found in real stained glass pieces.
Use Cases
-
Digital Art:Create stunning digital artworks that embody the beauty of stained glass.
-
Interior Design: Design virtual stained glass elements for architectural visualization.
-
Graphic Design: Incorporate stained glass aesthetics into logos, branding, and marketing materials.
-
Personal Projects: Craft unique, personalized gifts or decorations with a stained glass theme.
Other Popular Models
sdxl-img2img
SDXL Img2Img is used for text-guided image-to-image translation. This model uses the weights from Stable Diffusion to generate new images from an input image using StableDiffusionImg2ImgPipeline from diffusers
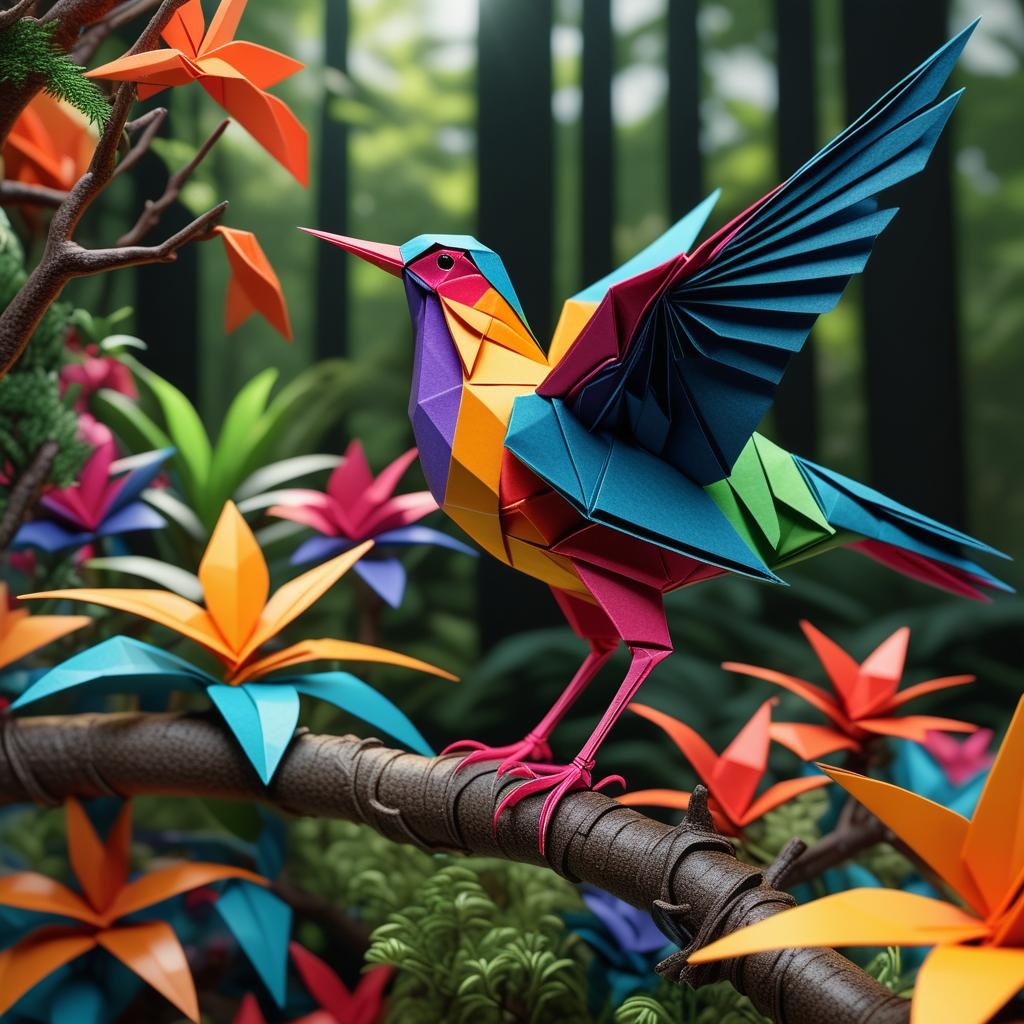
face-to-many
Turn a face into 3D, emoji, pixel art, video game, claymation or toy
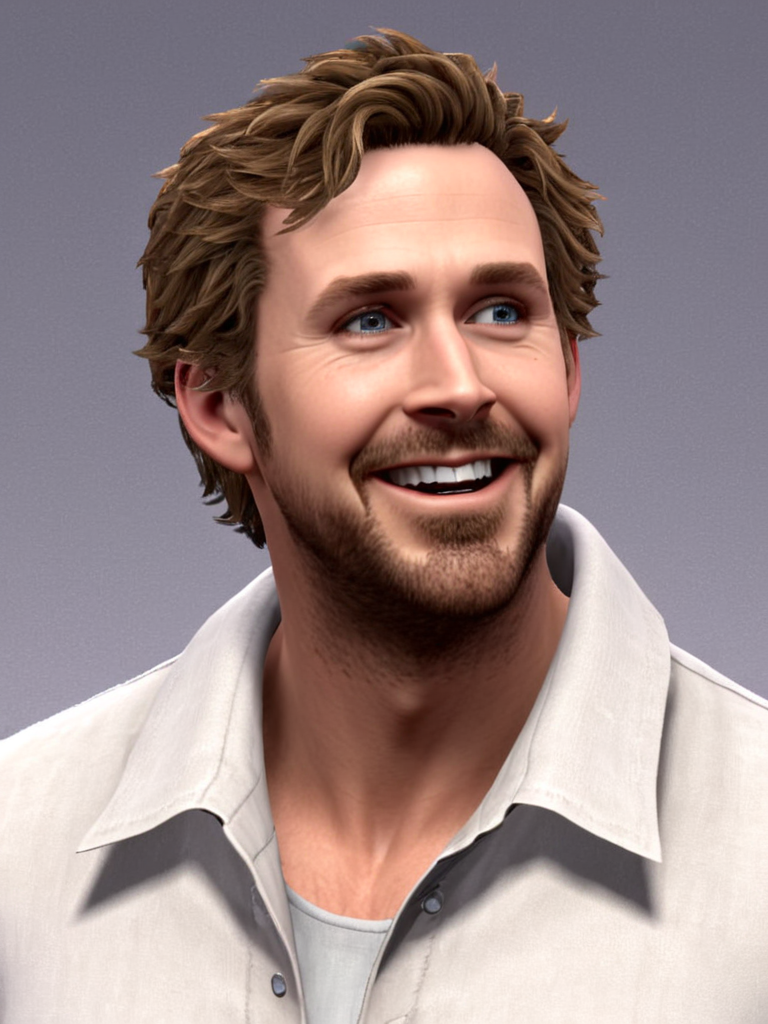
sd1.5-majicmix
The most versatile photorealistic model that blends various models to achieve the amazing realistic images.
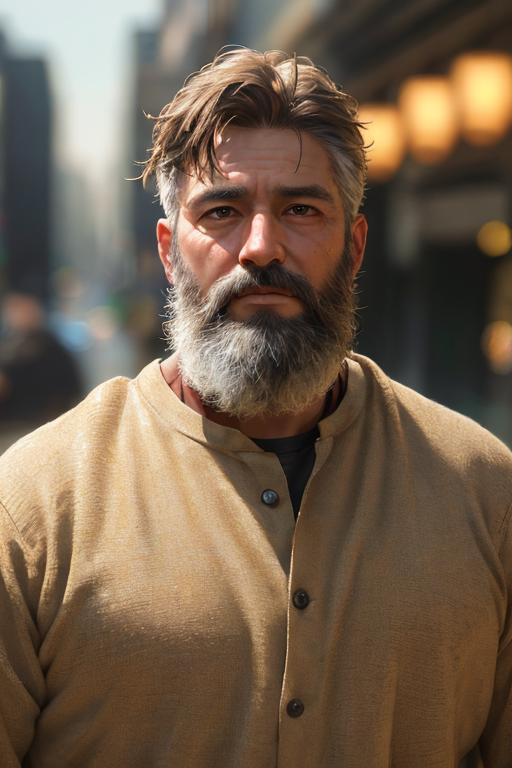
sd2.1-faceswapper
Take a picture/gif and replace the face in it with a face of your choice. You only need one image of the desired face. No dataset, no training
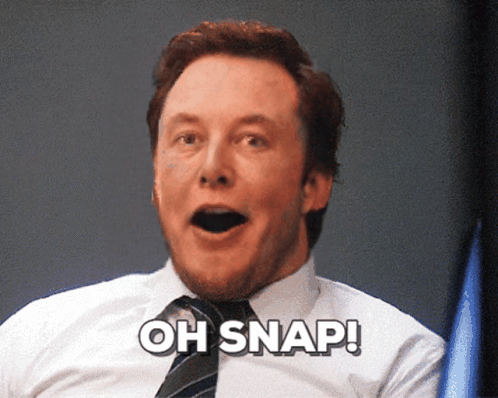